Qdrant Hybrid Search Combined with SQL Query Results
Apr 22, 2025
7 min read
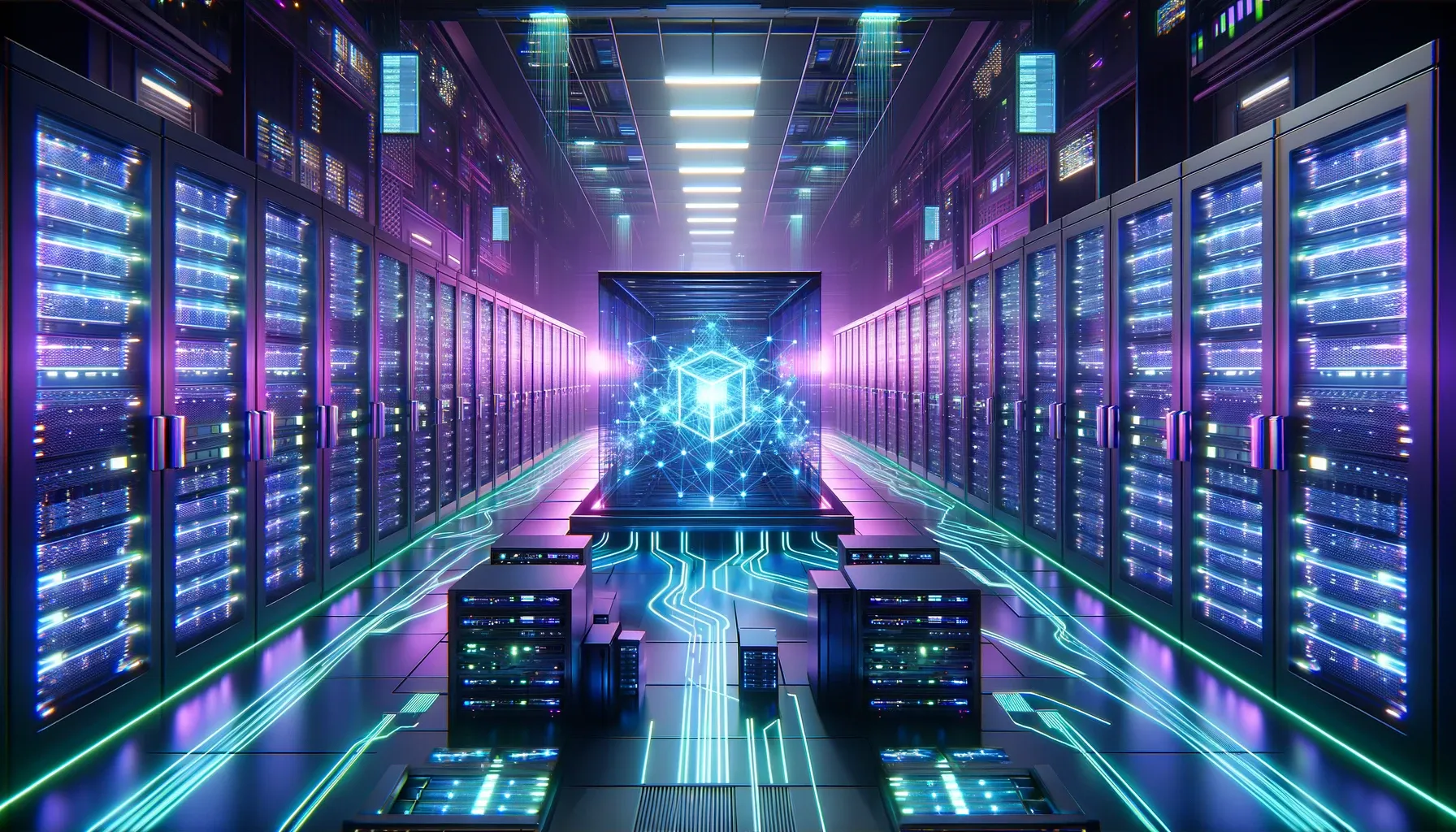
When developing AI-powered search systems, engineers often face a critical challenge: how to combine the contextual understanding of vector search with traditional database filtering. In this guide, we’ll explore how Qdrant’s hybrid search capabilities can be seamlessly integrated with SQL query results to create powerful constrained similarity searches.
My Favorite Vector Database: Qdrant
Qdrant has emerged as a leading vector database thanks to its:
- Native support for both dense and sparse vectors
- Efficient hybrid search capabilities
- Simple integration with Python ecosystems
- Production-ready performance characteristics
Its unique architecture enables simultaneous processing of multiple vector types while maintaining low latency - perfect for real-time applications.
FastEmbed: Lightweight Embedding Powerhouse
Qdrant’s FastEmbed library provides optimized model execution with:
- Minimal dependencies (no PyTorch/TensorFlow required)
- ONNX Runtime backend for hardware acceleration
- Support for popular models like all-MiniLM-L6-v2
- BM25 implementation for sparse vectors
This combination enables efficient embedding generation without sacrificing performance, crucial when working with large datasets.
The Hybrid Search Imperative
When Pure Semantic Search Fails
Consider this search scenario with programming language vs animal ambiguity:
document_contents = [ # The following contents are related to the Python programming language # But the word "Python" is not in the text "A versatile scripting language with dynamic typing and indentation-based syntax", "The preferred language for machine learning and data analysis", "Packages like NumPy and Pandas are essential for scientific computing", "Why __init__ and self are key concepts in this programming paradigm", "Django and Flask are popular frameworks for web development", 'def main(): print("Hello, World!")',
# The following contents are related to python snakes "Snakes like the reticulated python can grow over 20 feet long", "The reticulated python is the world's longest snake species",]
A pure semantic search for “Python” using dense vectors might prioritize programming content due to:
- Embedding model training bias towards technical content
- Semantic similarity clustering in vector space
- Lack of explicit keyword signals
This creates a problem for users searching for the animal - the zoologist in our example will get irrelevant programming results via dense vector search:
response = client.query_points( collection_name=COLLECTION_NAME, query=query_dense_embedding.tolist(), using=dense_vector_name,)
Printing the response.points
shows the following
id=5 version=0 score=0.5077583 payload={'sql_record_id': 5, 'content': 'def main(): print("Hello, World!")'} vector=None shard_key=None order_value=Noneid=2 version=0 score=0.4901533 payload={'sql_record_id': 2, 'content': 'Packages like NumPy and Pandas are essential for scientific computing'} vector=None shard_key=None order_value=Noneid=1 version=0 score=0.38752288 payload={'sql_record_id': 1, 'content': 'The preferred language for machine learning and data analysis'} vector=None shard_key=None order_value=Noneid=0 version=0 score=0.36844614 payload={'sql_record_id': 0, 'content': 'A versatile scripting language with dynamic typing and indentation-based syntax'} vector=None shard_key=None order_value=Noneid=4 version=0 score=0.35516733 payload={'sql_record_id': 4, 'content': 'Django and Flask are popular frameworks for web development'} vector=None shard_key=None order_value=Noneid=7 version=0 score=0.3404391 payload={'sql_record_id': 7, 'content': "The reticulated python is the world's longest snake species"} vector=None shard_key=None order_value=Noneid=3 version=0 score=0.29858643 payload={'sql_record_id': 3, 'content': 'Why __init__ and self are key concepts in this programming paradigm'} vector=None shard_key=None order_value=Noneid=6 version=0 score=0.2927005 payload={'sql_record_id': 6, 'content': 'Snakes like the reticulated python can grow over 20 feet long'} vector=None shard_key=None order_value=None
BM25: The Keyword Savior
BM25 (Best Match 25) solves this by:
- Calculating term frequency-inverse document frequency (TF-IDF)
- Prioritizing exact keyword matches
- Handling short queries effectively
- Working with sparse vector representations
In our example, BM25 would boost documents containing “python” while dense vectors handle semantic matches.
Reciprocal Rank Fusion (RRF): Merging Semantic and Keyword Results
RRF combines results from multiple search methods by:
- Getting ranked lists from each search type
- Assigning scores based on reciprocal ranks
- Merging results using formula:
1/(rank + k)
- Producing unified relevance ranking
This fusion preserves keyword matches while maintaining semantic relevance.
Implementing Hybrid Search in Qdrant
Model Configuration
# Configure dense vectors (semantic understanding)client.set_model( embedding_model_name="sentence-transformers/all-MiniLM-L6-v2", cache_dir="./fastembed-cache",)
# Configure sparse vectors (keyword matching)client.set_sparse_model( embedding_model_name="Qdrant/bm25", cache_dir="./fastembed-cache",)
After setting the embedding models in Qdrant, one can get the models later from the name-to-model dictionaries embedding_models
and sparse_embedding_models
:
from fastembed import TextEmbedding, SparseTextEmbedding
# Get the dense embedding modeldense_embedding_model: TextEmbedding = client.embedding_models[ "sentence-transformers/all-MiniLM-L6-v2"]
# Get the sparse embedding modelsparse_embedding_model: SparseTextEmbedding = client.sparse_embedding_models[ "Qdrant/bm25"]
Collection Creation
# Get vector configuration parametersdense_params = client.get_fastembed_vector_params()sparse_params = client.get_fastembed_sparse_vector_params()
# Create multi-vector collectionclient.create_collection( collection_name="demo-collection", vectors_config=dense_params, sparse_vectors_config=sparse_params,)
Data Insertion Strategy
For demonstration purpose, suppose each document only consists of an ID and a content field:
# The variable `document_contents` is the same as mentioned previously
documents = [ {"id": index, "content": document_content} for index, document_content in enumerate(document_contents)]
Insert into Qdrant:
# Generate combined embeddingsdense_embeddings = dense_embedding_model.embed(document_contents)sparse_embeddings = sparse_embedding_model.embed(document_contents)
# Create points with dual vectorspoints = [ models.PointStruct( id=doc["id"], vector={ dense_vector_name: dense.tolist(), sparse_vector_name: sparse.as_object(), }, payload={ # Associated the the record in SQL database "sql_record_id": doc["id"],
# Document content "content": doc["content"] } ) for doc, dense, sparse in zip(documents, dense_embeddings, sparse_embeddings)]
client.upsert(collection_name="demo-collection", points=points)
Note that calling the methods .tolist()
and as_object()
is required to convert numpy arrays and SparseEmbedding
object to Python data structures so that they can be passed to the Qdrant API.
Hybrid Query Execution
response = client.query_points( collection_name="demo-collection", prefetch=[ # Dense vector search models.Prefetch( query=query_dense_embedding.tolist(), using=dense_vector_name, limit=10, ),
# Sparse vector search models.Prefetch( query=query_sparse_embedding.as_object(), using=sparse_vector_name, limit=10, ), ], query=models.FusionQuery(fusion=models.Fusion.RRF),)
This returns results combining semantic matches from dense vectors and keyword hits from BM25.
id=7 version=0 score=0.64285713 payload={'sql_record_id': 7, 'content': "The reticulated python is the world's longest snake species"} vector=None shard_key=None order_value=Noneid=5 version=0 score=0.5 payload={'sql_record_id': 5, 'content': 'def main(): print("Hello, World!")'} vector=None shard_key=None order_value=Noneid=6 version=0 score=0.44444445 payload={'sql_record_id': 6, 'content': 'Snakes like the reticulated python can grow over 20 feet long'} vector=None shard_key=None order_value=Noneid=2 version=0 score=0.33333334 payload={'sql_record_id': 2, 'content': 'Packages like NumPy and Pandas are essential for scientific computing'} vector=None shard_key=None order_value=Noneid=1 version=0 score=0.25 payload={'sql_record_id': 1, 'content': 'The preferred language for machine learning and data analysis'} vector=None shard_key=None order_value=Noneid=0 version=0 score=0.2 payload={'sql_record_id': 0, 'content': 'A versatile scripting language with dynamic typing and indentation-based syntax'} vector=None shard_key=None order_value=Noneid=4 version=0 score=0.16666667 payload={'sql_record_id': 4, 'content': 'Django and Flask are popular frameworks for web development'} vector=None shard_key=None order_value=Noneid=3 version=0 score=0.125 payload={'sql_record_id': 3, 'content': 'Why __init__ and self are key concepts in this programming paradigm'} vector=None shard_key=None order_value=None
As you can see, the documents related to the python snake make it to the top 3. The zoologist must be pleased now.
SQL Integration
SQL databases excel at:
- Complex conditional filtering
- Join operations across datasets
- Access control enforcement
- Transactional data management
By first filtering records via SQL queries, we create constrained search spaces for vector operations.
Implementation Pattern
- SQL Pre-Filtering:
# Get accessible document IDs from SQLallowed_ids = execute_sql("SELECT id FROM documents WHERE department = 'zoology'")
- Constrained Vector Search:
response = client.query_points( collection_name="demo-collection",
# Query filter query_filter=models.Filter( must=models.FieldCondition( key="sql_record_id", match=models.MatchAny(any=allowed_ids), ) ),
# Search settings...)
Real-World Use Cases
-
Role-Based Access Control:
- Filter documents based on user permissions
- Maintain security while enabling semantic search
-
Temporal Filtering:
- Search only within recent documents
- Combine “last 7 days” SQL filter with vector search
-
Multi-Tenancy:
- Scope search to tenant-specific data
- Maintain single collection architecture
The Ultimate Combination
By merging SQL’s filtering power with Qdrant’s hybrid search, we create systems that:
- Enforce business rules through SQL constraints
- Understand user intent via semantic search
- Respect keyword matches through BM25
- Combine results intelligently via RRF
This architecture supports complex requirements while maintaining search relevance - whether your users are zoologists searching for snakes or developers looking for Python frameworks.
# Final hybrid search with SQL constraintsresponse = client.query_points( collection_name="demo-collection",
# Query filter to get target documents query_filter=models.Filter( must=[ # Only points with sql_record_id of 1, 3, 5, 7 # in the payload are considered models.FieldCondition( key="sql_record_id", match=models.MatchAny(any=[1, 3, 5, 7]), ) ] ),
# Hybrid search prefetch=[ models.Prefetch( query=query_dense_embedding.tolist(), using=dense_vector_name, limit=10, ), models.Prefetch( query=query_sparse_embedding.as_object(), using=sparse_vector_name, limit=10, ), ],
# RRF query=models.FusionQuery(fusion=models.Fusion.RRF),)
The result? Precise, secure, and context-aware search experiences that leverage the best of both worlds - structured data management and AI-powered similarity search.
Print the response.points
:
id=5 version=0 score=0.8333334 payload={'sql_record_id': 5, 'content': 'def main(): print("Hello, World!")'} vector=None shard_key=None order_value=Noneid=7 version=0 score=0.75 payload={'sql_record_id': 7, 'content': "The reticulated python is the world's longest snake species"} vector=None shard_key=None order_value=Noneid=1 version=0 score=0.5833334 payload={'sql_record_id': 1, 'content': 'The preferred language for machine learning and data analysis'} vector=None shard_key=None order_value=Noneid=3 version=0 score=0.4 payload={'sql_record_id': 3, 'content': 'Why __init__ and self are key concepts in this programming paradigm'} vector=None shard_key=None order_value=None
Comments 💬